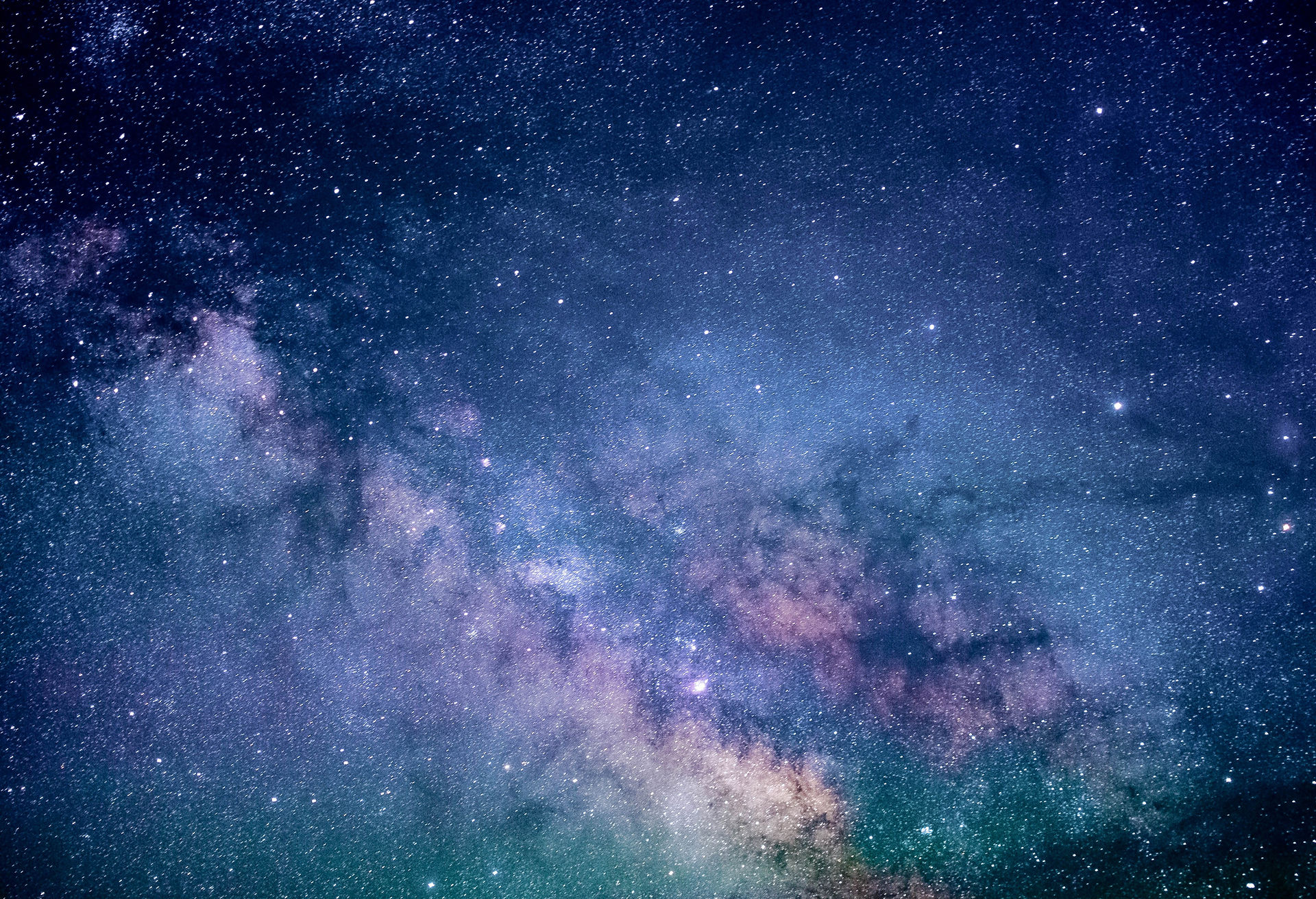
Sophia Zheng, Senior at American Heritage School Boca/Delray
Abstract
The purpose of this experiment was to utilize machine learning to analyze a dataset of migration patterns to determine places where humanitarian relief is most needed after a natural disaster, thus increasing the efficiency of aid distribution. The hypothesis was that if machine learning is implemented to analyze a dataset of population patterns, then it can predict migration patterns after disasters with a higher accuracy than the regular human researcher can and it can increase the efficiency of aid distribution during disaster crises. A dataset from Kaggle titled “Human Mobility During Natural Disasters” was analyzed using Weka software. The dataset consisted of the geographic coordinates of tweets during Typhoon Wipha. Three models were built by leveraging principal component analysis (PCA): K-means, Density based clustering, and Farthest First. As seen by the mappings, there were distinct hotspots of certain coordinates so these models could be used to analyze future test inputs, so the hypothesis was supported in that a machine learning model could be built on the available data to test further data. However, further test data was unavailable so the hypothesis was not supported in regards to test accuracy. The hotspots were concentrations of correctly identified clusters, which is what the focus was. By creating various machine learning models based on PCA, the researcher can help identify where hotspots of aid would be most necessary after or during a natural disaster which increases the efficiency of humanitarian aid.